A novel bioinformatics method from the Hovig group is published in a prestigious proteomics journal Mapping the cooperation between life's "molecular machines"
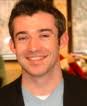
Eivind Hovigs bioinformatics group have taken the understanding of signaling systems in the cell one step up from the individual proteins, to describing communication of protein complexes. They have now published a novel computational method that predicts cooperation between pairs of protein complexes (often called "molecular machines") in cells. The first author of the work is Trevor Clancy at the department of Tumor Biology (photo), and is to be published in the prestigious journal Molecular and Cellular Proteomics (impact factor 7.39). Their computational method developed with collaborators Einar Andreas Rødland and Ståle Nygard now allows researchers to predict interactions between protein complexes. The mapping of these cooperative interactions using their procedure may be key to unraveling cellular organization, and to understand how this organization malfunctions in diseases such as cancer.
Mapping the cooperation between life's molecular machines
Researchers within professor Eivind Hovigs bioinformatics group at the Oslo University Hospital are taking the understanding of signaling systems in the cell one step up from the individual proteins, to describing communication of protein complexes. They have now published a novel computational method in the journal of Molecular and Cellular Proteomics, that predicts cooperation between pairs of protein complexes (often called "molecular machines") in cells. These molecular machines dynamically form from groups of individual protein parts, and once assembled, carry out specialized functions. Most of the biochemical activity that underpins cellular life can be credited to the functions of proteins and their aggregate molecular machines. In order to perform their biochemical alchemy: these molecular machines often cooperate with each other in a temporal and a location specific manner. Understanding and mapping these cooperative interactions will be key to unraveling cellular organization, and to understand how this organization malfunctions in diseases such as cancer. Unfortunately, mapping these interactions on a large scale is almost impossible using current experimental techniques: the assembly of these complex molecular machines and their inter-complex interactions are often very transient, unstable and therefore difficult to probe using conventional wet lab approaches.
Fortunately, in recent years, thanks to advances in proteomic experimental lab methods, many of the individual components that form these complex molecular machines have been identified. Although these descriptions are far from complete, they have proven to be sufficiently detailed to allow Clancy and colleagues at the Hovig lab to devise a computational strategy to predict and distinguish between these physical intra-complex and inter-complex interactions. The strategy involved the use of bioinformatics, the integration of molecular databases, and a customized statistical procedure that accounted for key biological features of molecular machines. Devising computational strategies which can accurately identify these types of interactions is hugely challenging, due to the numerous sources of noise that can confound the task. . However, the computational method developed by Clancy and co-workers allows researchers to reliably predict some of these cooperative interactions using a computer program. Their methodology has been tested against a list of manually selected control interactions identified previously in yeast, and the approach has been shown to successfully predict approximately half of these cooperations. Additionally, the output from their approach was found to be consistent with the current understanding of how the cell is organized, both functionally and spatially at a molecular level. While a more complete description of the individual components that form these complex molecular machines and further refinements to the method will be required to generate a complete map of the cells biochemical machinery, the tool has performed well, and represents a very promising start to this nascent field.
In this age of big-data science, with its rapid accumulation of molecular data from high throughput experiments, it is crucial to complement this progress with new computational methods, to prevent researchers from drowning in the sea of data, and to facilitate biological interpretation. Their method is one step towards organizing this biological complexity, and towards creating a more complete map of the cell.
In response to a request from "Molecular and Cellular Proteomics" of submitting a potential cover figure, the authors have sent the following illustration, that might end up on the cover of the May issue:
![]() |
The figure depicts a bioinformatics method to predict interactions between protein complexes. The method uses gold standard databases of protein complexes and a customized statistical procedure to capture interactions between the complexes. When a network of these complex-complex predictions were analyzed, it was found that communities of the complexes were consistent with the current understanding of cell organization. Such bioinformatics analyses may serve as a guide to the discovery of novel functional cellular relationships. |
Links:
The article:
Predicting physical interactions between protein complexes
Trevor Clancy, Einar Andreas Rødland, Ståle Nygard, Eivind Hovig
MCP Papers in Press. Published on February 25, 2013 as Manuscript O112.019828
Affiliations:
Trevor Clancy and Eivind Hovig : Department of Tumor Biology, Institute for Cancer Research, The Norwegian Radium Hospital, Oslo University Hospital, Oslo, Norway
Eivnd Hovig: Biomedical Research Group, Department of Informatics, Faculty of Mathematics and Natural Sciences, University of Oslo, Oslo, Norway
Einar Andreas Rødland: Center for Cancer Biomedicine, University of Oslo, Oslo, Norway
Ståle Nygard: Bioinformatics Core Facility, Institute of Medical Informatics, University of Oslo, Oslo University Hospital, Oslo, Norway
Eivind Hovig: Institute of Medical Informatics, The Norwegian Radium Hospital, Oslo University Hospital, Oslo, Norway
MCP - Molecular Celle Proteomics (journal impact factor 7,39)
Home page of Eivind Hovig's group